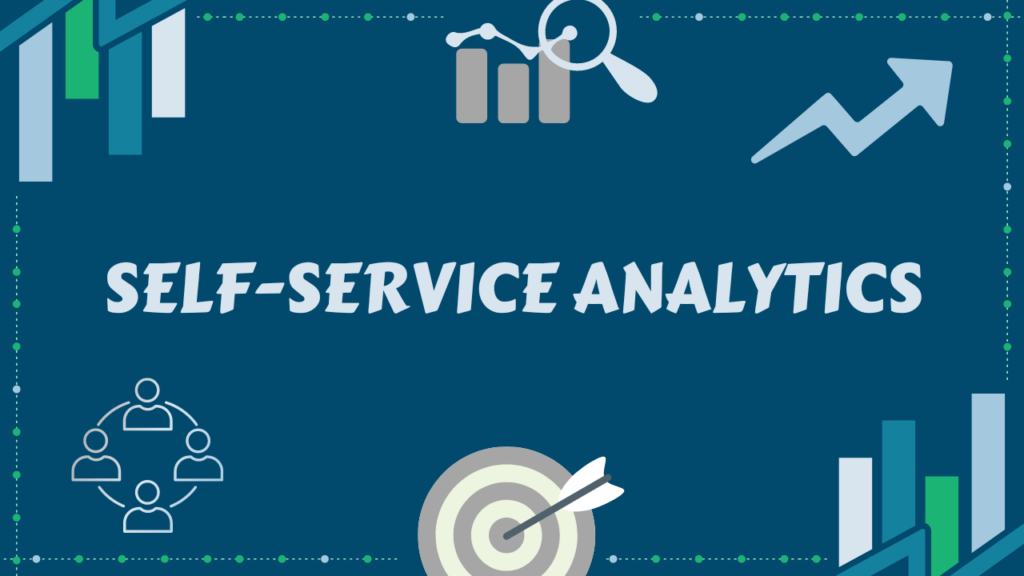
What is self-service analytics?
Self-service analytics a new way of doing analytics where business users are enabled to access data to generate insights on their own.
In a traditional analytics process, requests are handled by a centralized data/BI team.
With self-service analytics, the IT or centralized data team works on more complex tasks because business users can perform ad-hoc requests and other tasks that were previously done by the data team.
Business users are happier because they don’t have to wait for days or months to create a simple data report.
The centralized data team is happier because they have less on their plate and they can focus on high priority tasks.
But self-service analytics is NOT free.
What do you need for successful self-service analytics?
Here’s a few prerequisites for successful self-service analytics.
1. Data governance
Without good data governance, self-service analytics will just create a mess of data silos.
Everyone should know where to go to get information like these:
- What data assets can be used for what purpose?
- What are the limitations of each data asset?
- What’s the source of each data asset?
- Who do we need to contact about a data asset?
- Who’s responsible for data quality of each data asset?
Another thing is to implement the standardization of business metric definitions across the organization.
Business users creating their own solutions meaning there will be many more reports/dashboards.
There will likely be users that use different definitions for a metric if there is no governance.
Making sure everyone is using the consistent metric definitions is crucial in order to avoid making business decisions based on inaccurate metrics.
2. Self-service analytics tool(s)
You need at least a tool to perform queries and to generate reports/dashboards to gain insights.
Popular BI tools like Tableau, Power BI, Qlik, and Looker come to my mind for this.
Tools tailored towards self-service analytics such as Retool and Sigma may be a good use case as well.
Depending on each tool, the level of technical expertise required is different.
Choosing one that fits your organization’s needs is crucial.
A few things to look out for when choosing a tool for self-service analytics:
- Ease of use
- Capability to implement complex business logics
- Integrations with other tools such as Excel
- Swift response and support from the vendor
3. Technical training and support
Technical trainings and support are necessary for self-service analytics because business users still need to learn how to use the chosen tool(s).
Although there are and will be power users, you can assume many of your business users don’t have a technical background.
Ideally, they use it with best practices to use resources and create their data solutions the best way possible.
Here, the centralized data team comes into play.
They work as enablers and provide guidance and resources as subject matter experts for business users to execute analytics efficiently.
In short, without technical support, business users won’t be able to accomplish as much.
With the right technical resources, combined with domain knowledge of business users, your organization can accomplish much more.
Conclusion
By now, you can see that with self-service analytics implemented successfully, you’d increase the level of data literacy and data driven decision making culture in your organization.
And that leads to greater ROI (return on investment) on your analytics strategy.
I personally see self-service analytics as the future of analytics and business intelligence.
Why not enable business users to DIY (do it yourself) when that makes things easier for everyone?